By 1Nurul Asni Mohamed, 2M Aswadi Ton Alias and 3Izzatdin A Aziz. 1asnimo@petronas.com, 2aswadi_ton@petronas.com, Material Corrosion and Inspection, PETRONAS 3 izzatdin@utp.edu.my, Center for Research in Data Science (CeRDaS), Universiti Teknologi PETRONAS
Background
Currently in the oil and gas industry, corrosion rate predictions are generated via mathematical equations or correlations as an outcome from laboratory testing and analyses. The advancement of IoT and sensor technology has seen the implementation of wireless sensors such as nonintrusive wall thickness measurement sensors and temperature sensors being installed on piping surfaces throughout the refinery. These sensors generate high-frequency data streams in the masses. Statistical and mathematical equations, as well as correlation and forecasting work via sampling data, limits the use of inputs generated from big data produced by sensors and lab results. However, only recently has the oil and gas industry started to adopt machine learning algorithms as a tool for predicting future corrosion rates, following in the footsteps of other fields such as medical, banking, and the environment which have long utilised machine learning.
Before we delve deeper into the topic of the application of artificial intelligence in corrosion data sets, it is crucial to emphasise the significance of having a corrosion management system in place that covers all stages of an asset’s life cycle, from design and construction to operation and decommissioning, as shown in Figure 1.
Corrosion study is conducted during the Risk Based Inspection (RBI) development, ideally before the operational stage of a facility. This gives the added advantage of ensuring inspection and corrosion monitoring plans are in place upon starting up so that corrosion engineers can focus on system performance during operation. During the corrosion study, potential damage mechanisms that can be present are listed, which can mainly be non-age related and age-related mechanisms.

Degradation Mechanisms
Non-age-related damage mechanisms are failures of equipment or piping due to cracking, which makes it difficult to predict its useful life in operation and therefore are not designed for online monitoring. The mechanisms need to be addressed via:
- Optimum material selection
- Proper material specification
- Reliable manufacturer track record
- Materials manufacturing inspection and testing documented evidence
- Construction code compliance (e.g., post-weld heat treatment)
- Appropriate non-destructive examination technique & effective coverage
- Company best practices and lessons learnt.
Some examples of non-age-related mechanisms taken from API 571 are listed in Table 1.

An example of a non-age-related mechanism explained is Stress Relaxation Cracking (SRC) where it is caused by the accommodation of strain due to both carbide/nitride/intermetallic compound precipitation within grains inhibiting dislocation movement and the formation of precipitation free zone along the M23C6 carbide at grain boundary during stress relaxation process of welding at temperatures between 550°C and 750°C (during PWHT). SRC occurs at the chromium depleted zone when the accommodation of strain is beyond creep fracture ductility, as represented in Figure 2. Figure 3 shows the failure of an SA312 SSTP304H Steam Reformer Inlet Pigtail due to SRC. In this case, compliance to fabrication specification requirements, summarised in Figure 4, are critical factors to mitigate SRC which cannot be monitored via conventional corrosion monitoring techniques. Proper solution annealing should reveal homogeneous grain structure throughout a thin material. However, Figure 5 from scanning electron microscopy captured the presence of intermetallics whilst microscopy from Figure 6 revealed the presence of intergranular cracks with fine carbides dispersed. This evidence strongly suggests SRC to be the mechanism of failure.

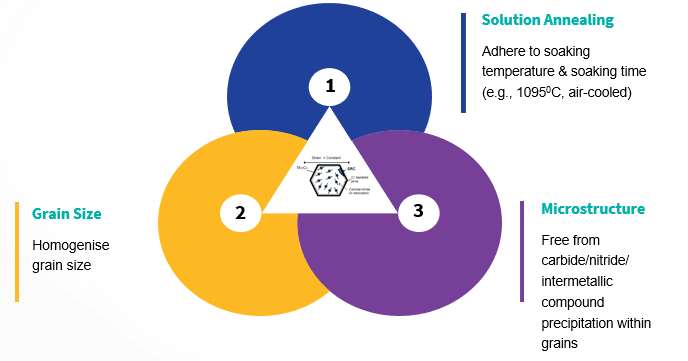
Age-related damage mechanisms on the other hand are failures of equipment or piping due to general or localised metal loss where its useful life in operation can be predicted. The mechanisms need to be addressed via:
- Optimum material selection
- Proper material specification
- Materials testing evidence
- Construction code compliance
- Company best practices (e.g., location, corrosion allowance)
- Effective online corrosion monitoring
- Predictive corrosion program
- Materials performance assessment
- Company lessons learnt and best practices should also be taken into consideration in addition to international standards guidelines.
Some examples of age-related mechanisms taken from API 571 are listed in Table 2.
Table 1. Non-age related mechanisms (API 571)
Cracking (Non-Age-Related) | |
1 | 485C Embrittlement |
2 | Amine Stress Corrosion Cracking |
3 | Ammonia Stress Corrosion Cracking |
4 | Brittle Fracture |
5 | Carbonate Stress Corrosion Cracking |
6 | Caustic Stress Corrosion Cracking |
7 | Chloride Stress Corrosion Cracking |
8 | Corrosion Fatigue |
9 | Creep and Stress Rupture* |
10 | Decarburisation* |
11 | Dissimilar Metal Weld Cracking |
12 | Ethanol Stress Corrosion Cracking |
13 | Graphitisation* |
14 | High Temperature Hydrogen Attack |
15 | Hydrofluoric Acid SCC of Nickel Alloys |
16 | Hydrogen Embrittlement |
17 | Hydrogen Stress Cracking in HF Acid |
18 | Liquid Metal Embrittlement |
19 | Mechanical Fatigue inc Vibration Induced Fatigue |
20 | Nitriding |
21 | Polythionic Acid Stress Corrosion Cracking |
22 | Stress Relaxation Cracking (Reheat Cracking) |
23 | Short Term Overheating Stress Rupture (inc Steam Blanketing) |
24 | Sigma Phase Embrittlement |
25 | Strain Aging |
26 | Temper Embrittlement |
27 | Thermal Fatigue |
28 | Thermal Shock |
29 | Titanium Hydriding |
30 | Wet H2S Damage (H2 Blistering/HIC/SOHlC/SSC) |
Table 2. Age-related mechanisms (API 571)
Thinning (Age-Related) | |
1 | Amine Corrosion |
2 | Ammonium Bisulphide Corrosion |
3 | Ammonium Chloride and Ammonium Hydrochloride Corrosion |
4 | Aqueous Organic Acid Corrosion |
5 | Atmospheric Corrosion |
6 | Boiler Water and Steam Condensate Corrosion |
7 | Brine Corrosion |
8 | Carburisation |
9 | Caustic Corrosion |
10 | Cavitation |
11 | CO2 Corrosion |
12 | Concentration Cell Corrosion |
13 | Cooling Water Corrosion |
14 | Corrosion Under lnsulation |
15 | Dealloying |
16 | Erosion_Erosion Corrosion |
17 | Flue Gas Dew Point Corrosion |
18 | Fuel Ash Corrosion |
19 | Galvanic Corrosion |
20 | Gaseous Oxygen Enhanced Ignition and Combustion |
21 | Graphitic Corrosion of Case Irons |
22 | High Temperature H2_H2S Corrosion |
23 | Hydrochloric Acid Corrosion |
24 | Hydrofluoric Acid Corrosion |
25 | Metal Dusting |
26 | Microbiologically Influenced Corrosion |
27 | Naphthenic Acid Corrosion |
28 | Oxidation |
29 | Oxygenated Process Water Corrosion |
30 | Phenol (Carbolic Acid) Corrosion |
31 | Phosphoric Acid Corrosion |
32 | Refractory Degradation |
33 | Soil Corrosion |
34 | Sour Water Corrosion (Acidic) |
35 | Spheroidisation |
36 | Sulphidation |
37 | Sulphuric Acid Corrosion |

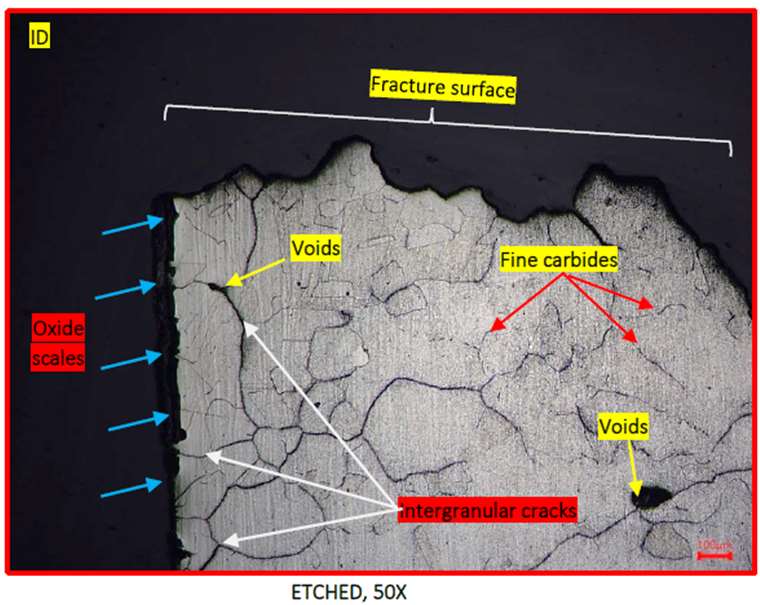
Artificial intelligence via machine learning algorithm modelling
This article will explain the steps taken to employ artificial intelligence via machine learning algorithms to predict corrosion rates for thinning mechanisms. To start, critical corrosion groups are selected based on domain experts’ experiences, internal lessons learnt and most importantly, the availability of corrosion rate monitoring sensors, which is the machine learning model’s target value. In order for the algorithm to be developed, only corrosion groups with online corrosion rate sensors and corrosion probes are selected to enable continuous data assessment.
Overview of i-CoRPA machine learning framework
Throughout the refinery and petrochemical complex, there are slightly over 1000 online corrosion monitoring sensors and 90 corrosion probes strategically located at areas for the purpose of monitoring general metal loss. The material of construction for these locations is carbon steel. In order to effectively manage corrosion, a machine learning modelling programme was developed, known as the Intelligent Corrosion Rate Predictive Analytics (i-CoRPA), incorporating Integrity Operating Windows (IOWs) from identified degradation mechanisms and correlating with available corrosion rate values to generate a future predicted corrosion rate. The i-CoRPA system’s framework is represented in Figure 7. Although the i-CoRPA system’s framework is developed based on the case studies and dataset from this downstream facility, the whole system is developed to be modular and pluggable to be implemented in other facilities with sensors and online data streaming technology. I-CoRPA is trained with more than 7 million data points gathered over 6 months period of data acquisition process. These historical datasets are cleansed from bad values, inconsistent readings, and imbalance categories prior as input into customised machine learning models for predictions.
In this study, the facility relays all sensors and lab data into a centralised recording system known as PI. I-CoRPA receives online data stream from the PI system and performs real-time data and batch data such as IOW data are pre-processed to handle missing values, bad values, and inconsistent data. Pre-processed data are then autonomously sent to the AI Model for prediction. Further explanation on the study and development of the AI model is detailed out in subsequent sections in the article.

Data transformation and pre-processing
As part of the corrosion study assessment, Integrity Operating Windows (IOW) are defined for key parameters that can affect the probability and progression rate of a particular damage mechanism. IOW may generally fall into two categories i.e., chemical parameters or physical parameters and may be obtained from online analysers, local indicators or process sampling. Examples of chemical parameters include pH and concentration of corrodent whereas physical parameters are those that are not chemical in nature such as operating pressure and temperature. Excursion to any of the IOW will require a timely response to bring the parameter back within the acceptable limit.
Data preprocessing is a step that involves transforming raw data so that issues owing to the incompleteness, inconsistency, and/or lack of appropriate representation of trends are resolved so as to arrive at a data set that is in an understandable format6. The goal of data preprocessing is to clean and generate a data set that can simplify the process while performing feature engineering and model training stages. Some of the methods that can be applied under data preprocessing include:
- Pivot – when data that is extracted from the database as a “row-format” are converted into “columnar-format” through a process called pivoting to prepare the data for the next step.
- Trim – involves the removal of observations without target “y” value or in this case corrosion rate.
- Reindex – applied to address a variety of data to fix the problem during which a discontinuity of time range exists in any of the data set producing gaps.
- Bad Tags Removal – is also known as noisy data, meaningless data that cannot be interpreted by machines. It can be generated due to faulty data collection, data entry errors etc.
In addressing the presence of too many parameters within a corrosion group that contributes to corrosion, we will need to figure out the optimum way forward to identify the best features and data characteristics to be selected. There is no single solution to this problem, hence, feature engineering is considered an “art” where the study of parameter correlation is to be performed to obtain the most suitable combination. Under feature engineering, feature selection is carried out before feeding the data to a predictive model to remove unnecessary features which can lead to undesired longer model processing time.
One important step under feature engineering is handling missing values. In any real-world data set, there are always a few null values. No model can handle these NULL or NaN values on its own. Very often, standard approaches to solving this problem do not exist, because the approaches largely depend on the context and nature of the data. One easy way to solve this problem is to simply ignore or delete rows that lack data measurements by deleting them from the analysis. However, this method may not be effective due to information loss. Therefore, we can analyse methods to fill in the missing values which will yield a high accuracy as output of the corrosion rate prediction modelling. These methods may involve data imputation via mean/mode/median approximation, regression and forecasting algorithms such as K-Nearest Neighbour and Multiple Imputation by Chained Equations (MICE). The corrosion data set encountered contains input features that can be labelled originating from Integrated Operating Window parameters such as dewpoint temperature, chloride ions, and output such as corrosion rate. Therefore, the supervised learning algorithm is applicable.
Common algorithms in supervised learning include logistic regression, naive bayes, support vector machines, artificial neural networks, and random forests. The final step of the machine learning modelling is the model validation. The predicted corrosion rates will be verified with actual thickness measurement at site.
Table 3. An example of model training showing model accuracy and time taken for processing.
Model | Accuracy (%) | Fit time (s) |
LightGBMXT_BAG_L1 | 84.55 | 49.14 |
LightGBMXT_BAG_L1_FULL | 84.41 | 5.05 |
WeightedEnsemble_L2 | 83.83 | 28.54 |
LightGBM_BAG_LI | 83.72 | 27.30 |
RandomForestMSE_BAG_L1_FULL | 83.51 | 0.93 |
RandomForestMSE_BAG_LI | 83.51 | 0.93 |
WeightedEnsemble_L2_FULL | 83.14 | 4.53 |
LightGBM_BAG_L1_FULL | 82.85 | 3.29 |
RandomForestMSE_BAG_L2 | 81.69 | 79.11 |
LightGBMXT_BAG_L2 | 81.46 | 113.65 |
Model training and scoring
To date, we have covered 30 process units which include different trains, and 120 corrosion groups selected from a total of about 3800 corrosion groups for the whole facility. 700 customised machine learning models were developed. Success is defined by best highest accuracy (>80%) with an optimum model run time. When accuracy is lower than expected, more often than not, the data set volume is lacking. Table 3 represents an example of model training output with percentage accuracy and time taken to process the model. Ideally, the best model will produce the highest output accuracy within the shortest processing time. However, sometimes a compromise will be reached to select a relatively high accuracy output, which is still above the threshold limit set of 80 percent, with a reasonably low processing time.
In this study, as many as 10 tree-based and boosting based AI algorithms were implemented onto the sensor and IOW data. This category of algorithms was selected due to its high-speed processing capability when dealing with high-frequency real-time data. Tree based algorithms are supervised learning paradigms which empower predictive models with high accuracy, stability, and ease of interpretation. Tree based models are unlike linear models, they are able to map nonlinear correlations very well and are adaptable to solving both regression and classification problems.
Figure 8 is one of the many examples representing a relatively high model accuracy when comparing actual to predicted corrosion rate. The figure also shows that the model is able to predict corrosion rate 27 days ahead at high accuracy. However, like many AI prediction models, the accuracy reading is expected to drop as the predicted period is extended further. However, this can be improved with more data with significant anomalous readings prepared for the model to learn. This should enrich the data by improving the labels for the algorithm to learn. The R2 measures at 92.57%, which indicates that the actual and predicted corrosion rate values are highly correlated, and the model is more than acceptable to be implemented. The highest accuracy reads at 81.8% with error measures at 18.82%. These accuracy and error values can be further improved as the system progresses and more real-time data are fed as input into the AI model to learn, hence making the model smarter and more efficient.
Fine-tuning of the models will be a continuous autonomous process and with more streaming data being fed into the model, the accuracy of the corrosion rate is also expected to be increased. Hyperparameter of the algorithm will be further calibrated against the results obtained, in which will produce better accuracy in a positive feedback manner. In conclusion, the main success of this machine learning initiative is enhanced corrosion rate monitoring via corrosion rate forecasting especially for such a huge facility. In the event of excursion of the predicted corrosion rate, the parameter that affects the prediction can be precisely identified and proper actions can be executed ahead of time. This capability has allowed for a more focused response to any IOW excursion whereby higher priority will be given to those that will lead to unacceptable predicted corrosion rate.

Conclusion
To summarise, the application of data analytics via AI machine learning is getting more traction lately mainly due to the availability of big data and the capability to analyse and process the variety and volume of data within a short period of time, much unlike the traditional laboratory testing to predict corrosion rate. However, currently the prediction focus is on age-related mechanisms. In order to ensure that the AI machine learning approach is a success, the corrosion engineers are also trained on big data analytics such as the use of Python and other coding applications which upskills them to hybrid engineers. The ability to predict corrosion rates for selected critical process systems in a complex refinery and petrochemical facility enables corrosion engineers to make informed decisions in a proactive manner, reduce the tedious work of analysing data using traditional excel spreadsheets, and therefore increase their overall efficiency. Generally, it was found that work efficiency has increased by 80% compared to the previous analysis approach, which meant that more time can be channelled to other critical tasks.
References
1 | ANSI/API Recommended Practice 571 Damage Mechanisms Affecting Fixed Equipment in the Refining Industry, Third Edition March 2020. |
2 | Myrianthous, G. Supervised vs Unsupervised Learning. https://towardsdatascience.com/understanding-the-different-types-of-machine-learningmodels-9c47350bb68a (2021) |
3 | Soni, D. Understanding the Different Types of Machine Learning Models. https://towardsdatascience.com/understanding-the-different-types-of-machine-learningmodels-9c47350bb68a (2019) |
About this Featured Story
This Featured Story appeared in Stainless Steel World August 2023 magazine. To read many more articles like these on an (almost) monthly basis, subscribe to our magazine (available in print and digital format) – SUBSCRIPTIONS TO OUR DIGITAL VERSION ARE NOW FREE.
Every week we share a new Featured Story with our Stainless Steel community. Join us and let’s share your Featured Story on Stainless Steel World online and in print.